Comparison of deep learning techniques for the investigation of a seismic sequence: an application to the 2019, Mw 4.5 Mugello (Italy) earthquake
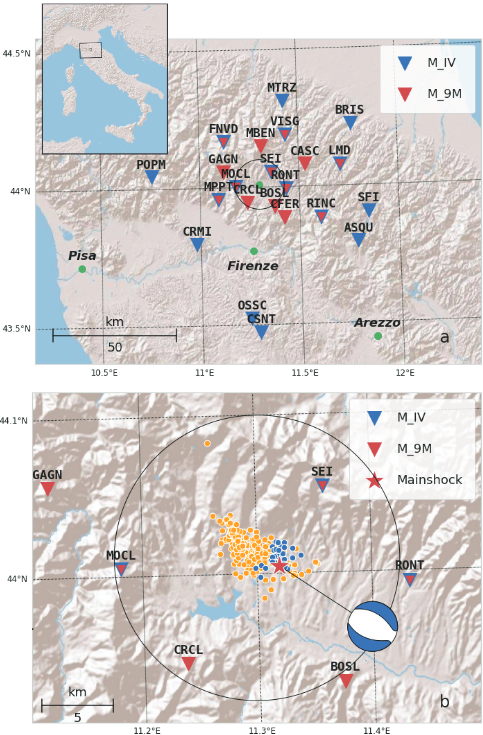
Cianetti S., R. Bruni, S. Gaviano, D. Keir, D. Piccinini, G. Saccorotti, C. Giunchi (2021).
JGR – Solid Earth, https://doi.org/10.1029/2021JB023405
Abstract
The increase of available seismic data prompts the need for automatic processing procedures to fully exploit them. A good example is aftershock sequences recorded by temporary seismic networks, whose thorough analysis is challenging because of the high seismicity rate and station density. Here, we test the performance of two recent Deep Learning algorithms, the Generalized Phase Detection and Earthquake Transformer, for automatic seismic phases identification. We use data from the December 2019 Mugello basin (Northern Apennines, Italy) swarm, recorded on 13 permanent and 9 temporary stations, applying these automatic procedures under different network configurations. As a benchmark, we use a catalog of 279 manually re-picked earthquakes reported by the Italian National Seismic Network. Due to the ability of deep learning techniques to identify earthquakes under poor signal-to-noise-ratio (SNR) conditions, we obtain: i) a factor 3 increase in the number of locations with respect to INGV bulletin; ii) a factor 4 increase when stations from the temporary network are added. Comparison between deep learning and manually picked arrival-times shows a mean difference of 0.02-0.04 s and a variance in the range 0.02-0.07 s. The improvement in magnitude completeness is ∼0.5 units. The deep learning algorithms were originally trained using datasets from different regions of the world: our results indicate that these can be successfully applied in our case, without any significant modification. Deep learning algorithms are efficient and accurate tools for data reprocessing in order to better understand the space-time evolution of earthquake sequences.
Devi effettuare l'accesso per postare un commento.